The topic of data spaces has gained increased traction in Europe and beyond. In this blog post, we will talk in a compact way about the history of the concept, its current definition, and the significant challenges that lie ahead for consolidating the idea in practice.
THEN
The concept of data spaces was introduced in 2005 [1] and originally referred to a data management approach for handling several data sources in the context of an organization. In this context, the meaning of the term “organization” ranges from, for example, enterprises and government agencies (large) to smart homes and personal devices (small). What these organizations have in common is that they need to handle data sources that are increasingly diverse, numerous, and interrelated, which entails many information management challenges, for example:
- Retrieval: How to search and query data across heterogeneous data sources?
- Rules: How to enforce policies? How to ensure integrity? How to manage naming conventions?
- Access: How to control data access? How to ensure availability?
- Evolution: How to manage not only the data, but also the metadata, over time?
As the authors emphasized, data spaces (back then spelled “dataspaces”) are not a data integration approach [1]. Data integration approaches require significant upfront effort, as they need semantic integration before any services can be provided. Instead, the focus of data spaces is put on data co-existence. Therefore, neither upfront integration nor exclusive control over the data is required to enable a data space.
The services needed to realize data spaces focus on base functionality over all data sources, support to all types of data within the data space, only on-demand integration efforts, and “best-effort“ results regarding data querying. The envisioned enabling services of data spaces included, for example, data cataloging, data search and query, data discovery, data monitoring, data updating, and event detection.
One important feature of the original definition of data spaces is that it focuses on data management needs in the context of an organization. Although the authors mention examples of organizations consisting of multiple (sub-)organizations (e.g., large science-related collaborations, multiple government agencies, etc.), they did not emphasize it. Interestingly, the opportunities for collaboration across organizations have become the focus of data space initiatives we see nowadays.
NOW
Many of the recent initiatives that have been working on how to realize data spaces have used a definition that does not underline the diversity, abundance, and relationships of the data sources, even though these characteristics still hold true. Instead, current definitions of data spaces as a data management approach give prominence to the existence of many stakeholders exchanging (or interested in exchanging) data. The main stakeholders in a data space are the data provider (who is typically, but not necessarily, the data owner) and the data consumer, followed by the data service provider [2]. Data providers provide raw data to the data space through catalogs, and data consumers benefit from either the raw data or processed data, which can be made available in a data space by the data service providers. Data service providers can be perceived as participants in the data space who process raw data and offer analytic results to data consumers.
Recent research [2] organizes key enablers of data spaces in three layers:
- Data connectors and infrastructure: This is the bottom layer, which provides the fundamental building blocks for data spaces. It tackles data management at a meta-level, i.e., it focuses on standardizing methods and formats to describe the data and the participants in a data space, to find them, to identify them, and to negotiate contracts based on agreed data usage policies.
- Data interoperability: On this middle layer, the goal is to enable data interoperability, i.e., to support the actual data exchange (not metadata exchange) among participants. This involves technological interfacing and data modeling measures. Syntactic and semantic interoperability among heterogeneous stakeholders must be achieved at this level.
- Data value: At the top, the data value layer leverages data interoperability to offer data-related services such as data analytics and data quality improvements (cleaning, feature selection, feature engineering, etc.).
To date, most existing data space initiatives have focused on data connectors and infrastructure, and there are at least two reasons for this. First, since they refer to the bottom layer, they provide a basis for the layers above. Second, given the increasing concerns about data sovereignty, data spaces have been envisioned as an opportunity to enable trust among participants engaged in data exchange. Two of the most prominent data space initiatives in Europe that have emphasized the data sovereignty aspect are IDS and Gaia-X. As described on the Gaia-X website, “Gaia-X enables and boosts the creating of data spaces through trusted platforms that comply with common rules, allowing users and providers to trust each other on an objective technological basis, to safely and freely share and exchange data across multiple actors.” [3] Concerning specifically the implementation of data connectors, the Eclipse Data Components (EDC) framework is a leading initiative. There, data space connectors are described as “logical gatekeepers that integrate into each [data space] participant’s infrastructure and communicate with each other” [4].
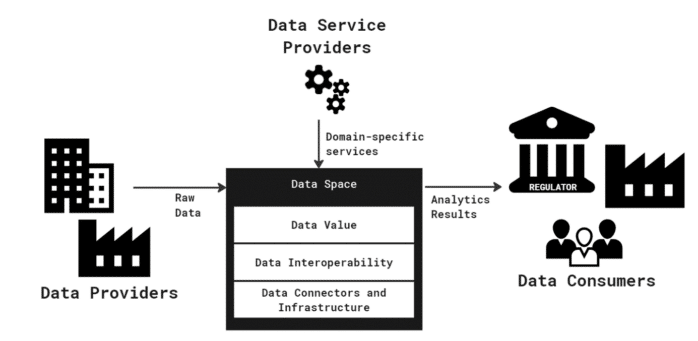
NEXT
The realization of functional data spaces depends on the availability of the three key enablers (data connectors and infrastructure, data interoperability, and data value). On the one hand, major initiatives have been set out to create trust frameworks to provide the means for data exchange at a meta-level (i.e., exchange about the participants and their data, not the data itself). Still, there is work to be done. The lack of common definitions for data spaces and connectors can impede interoperability even at the meta-level. For example, different policy settings languages have been used by different initiatives (e.g., ODRL, IDS Usage control language, LUCON), not to mention the incompatibility between different connector implementations.
While the work on data connectors and infrastructure for data spaces is not yet finished, the next challenges await around the corner: data interoperability and data value. Concerning data interoperability, initiatives related to semantic data, including the Next Generation Service Interfaces Linked Data [5] and the Smart Data Models Program [6], have contributed to the technological basis for new interoperable data and services. However, most existing data sources and related applications are legacy systems built independently, and semantic interoperability was not considered in their design. Therefore, the integration effort is very high. Matching data from different data sources is a multi-level challenge: As mentioned in [2], it is necessary to find semantic matchings between different ontologies (or vocabularies), between data schemes and ontologies, and between data entities in different organizations. NEC Lab has put forward one example of research work to address these matching challenges with their solution TrioNET [7].
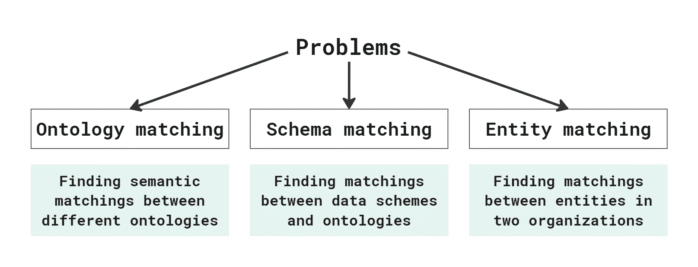
WRAP UP
- Then, data spaces were a data management approach that did not focus on data integration.
- Now, data spaces refer to data management with many stakeholders exchanging data in a trusted environment. Data spaces have three key enabling layers: connectors and infrastructure, data interoperability, and data value. The current development of most data spaces focuses on connectors and infrastructure.
- Next, data interoperability must be addressed. Pressing challenges refer to matching mechanisms and the compatibility of connectors.
Data spaces at IESE
We have been involved in the shaping of the current concept of data spaces for many years as participants in the International Dataspaces Association. In 2020, we researched the suitability of Digital Twins in the creation of a data space for the agricultural domain in the Fraunhofer lighthouse project COGNAC. More recently, we investigated the applicability of Gaia-X and EDC technologies to address the interoperability issues in the agricultural domain. We derived several use cases from this domain and compared what Gaia-X is currently able to support and how this takes interoperability into account. To further tackle the challenge of entity matching, we are currently exploring the use of LLMs to improve interoperability between systems of different organizations in the agricultural domain that adopt distinct standards.
Do you need conceptual or technological support to build or join a data space? Our experts in the Architecture-Centric Engineering and Security Engineering departments can help you. Get in touch with us!
References:
[1] Franklin, Michael, Alon Halevy, and David Maier. „From databases to dataspaces: a new abstraction for information management.“ ACM Sigmod Record 34.4 (2005): 27-33.
[2] Solmaz, Gürkan, et al. „Enabling data spaces: Existing developments and challenges.“ Proceedings of the 1st International Workshop on Data Economy. 2022.
[3] Gaia-X. “Gaia-X: a federated secure data infrastructure”. https://gaia-x.eu/. Accessed on March 4, 2024.
[4] Eclipse Dataspace Components. “Eclipse Dataspace Components”. https://eclipse-edc.github.io/docs/#/README. Accessed on March 4, 2024.
[5] ETSI Industry Specification Group (ISG). “Context Information Management (CIM); NGSI-LD API”. Technical Report. 2021. https://www.etsi.org/deliver/etsi_gs/CIM/001_099/009/01.04.01_60/gs_cim009v010401p.pdf. Accessed on March 4, 2024.
[6] Smart Data Model Program. “Smart data models”. https://smartdatamodels.org/. Accessed on March 4, 2024.
[7] NEC Laboratories Europe. “Data Ecosystems and Standards”. https://www.neclab.eu/research-areas/system-platforms-for-ai-and-des/des. Accessed on March 4, 2024.